Harnessing Big Data in Trading Decisions
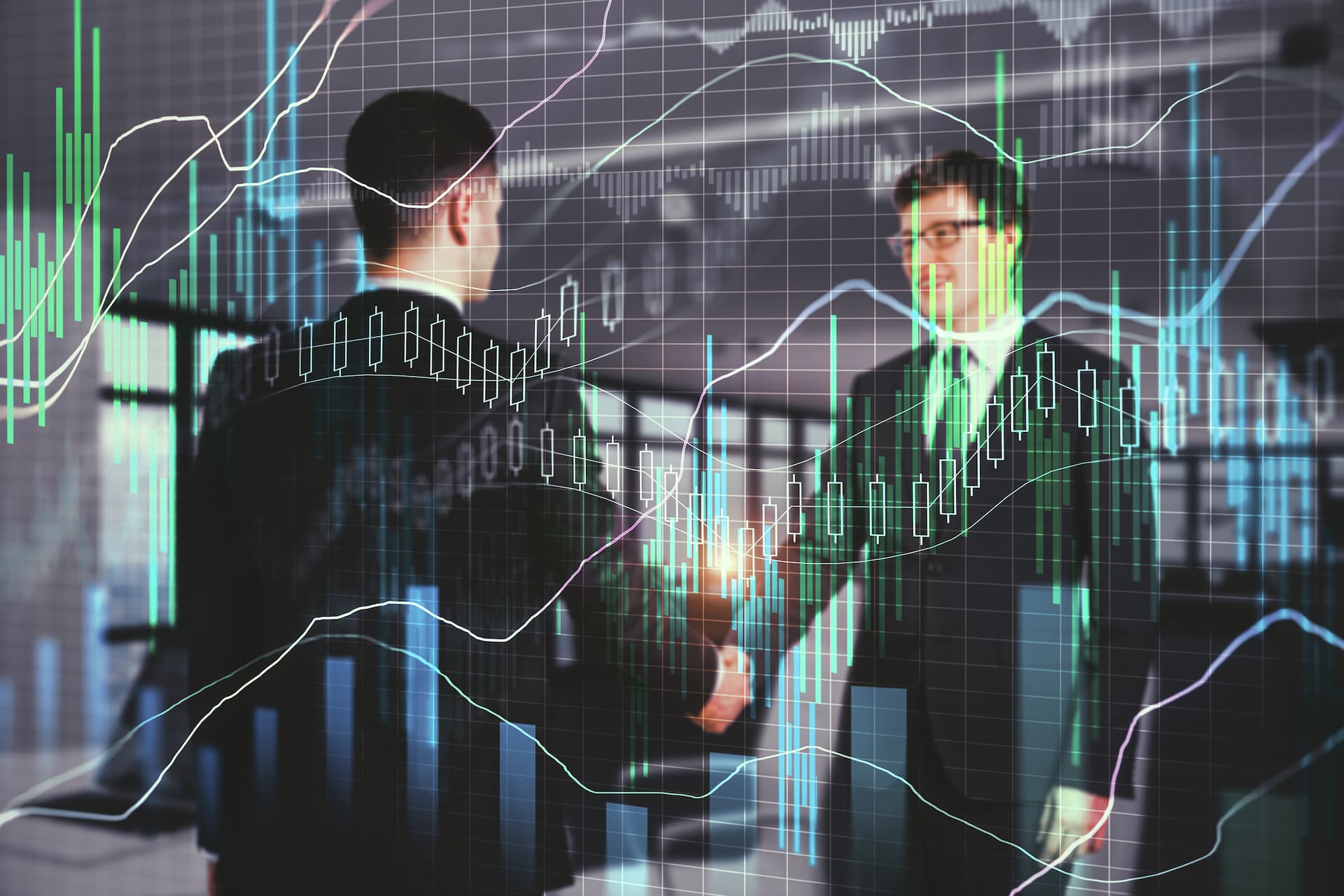
1. Understanding Big Data in Trading
Big data encompasses the massive volumes of structured and unstructured data generated by various sources. In trading, big data includes market data, news, social media, and other information that can provide critical insights. Explore how financial data improve trading strategies through analysis, predictions, and algorithmic trading.
What is Big Data?
Volume, Velocity, and Variety
Big data is defined by its volume (the sheer amount of data), velocity (the speed at which data is produced and processed), and variety (the different types of data). These characteristics make big data both challenging and advantageous for trading analysis.
- Volume: This refers to the vast amount of data generated, including trading volumes, market transactions, and historical data. For instance, daily trading activities across global markets generate enormous datasets that can be analyzed for patterns and trends.
- Velocity: The rapid rate at which data is generated, such as real-time price updates, economic news releases, and social media posts. Traders need tools that can process this data quickly to make timely decisions.
- Variety: The range of data types, including structured data (numerical data like stock prices) and unstructured data (textual data from news articles and social media). The diversity of data sources requires sophisticated methods for integration and analysis.
- Related Link: Learn more about the characteristics of big data.
Sources of Big Data in Trading
Market Data
Market data includes real-time and historical price quotes, trading volumes, and order book information. This data is fundamental for technical analysis and developing trading strategies.
- Example: Traders analyze historical price trends and real-time data to identify trading signals and execute trades. For instance, historical price patterns may be used to forecast future price movements and make informed trading decisions.
- Related Link: Explore market data sources.
News and Social Media
News and social media provide sentiment analysis and market-moving information. Tracking news events and social media trends helps traders gauge market sentiment and anticipate price movements.
- Example: By monitoring news headlines and social media sentiment, traders can assess how news events might impact market prices. For instance, positive news about a company may lead to a rise in its stock price, while negative news could lead to a decline.
- Related Link: Learn about social media sentiment.
Economic Indicators
Economic indicators, such as GDP, unemployment rates, and inflation data, influence market movements. Analyzing these indicators helps traders understand macroeconomic trends and their impact on financial markets.
- Example: Traders use economic indicators to predict market trends and adjust their strategies accordingly. For example, a rise in inflation might lead traders to anticipate changes in interest rates and adjust their investment positions.
- Related Link: Explore economic indicators.
2. Techniques for Harnessing Big Data
Effectively utilizing big data in trading requires specialized techniques and tools to process, analyze, and interpret the data.
Data Collection and Management
Data Aggregation
Aggregating data from multiple sources provides a comprehensive view of the market. Tools for data aggregation collect and consolidate information from various platforms, enabling traders to analyze a unified dataset.
- Example: Traders use data aggregation tools to combine market data, news, and social media insights into a single platform. This consolidated view helps in identifying trading opportunities and making more informed decisions.
- Related Link: Learn more about data aggregation.
Data Storage
Efficient storage solutions are crucial for managing big data. Cloud-based storage and data warehousing solutions offer scalable options for storing and accessing large datasets.
- Example: Cloud storage solutions allow traders to manage vast amounts of trading data efficiently. These solutions offer flexibility and scalability, accommodating the growing data needs of traders.
- Related Link: Explore data storage solutions.
Data Cleaning and Preprocessing
Data cleaning involves removing inaccuracies and inconsistencies from datasets, while preprocessing prepares data for analysis by transforming it into a suitable format.
- Example: Data cleaning may involve correcting errors in market data and removing irrelevant information. Preprocessing could include normalizing data formats and handling missing values to ensure accurate analysis.
- Related Link: Learn about data cleaning techniques.
Data Analysis
Statistical Analysis
Statistical methods help analyze and interpret big data. Techniques such as regression analysis, correlation analysis, and hypothesis testing identify patterns and relationships in the data.
- Example: Regression analysis can model the relationship between market variables and asset prices. This helps traders understand how different factors affect price movements and develop more accurate trading predictions.
- Related Link: Explore statistical analysis techniques.
Machine Learning and AI
Machine learning algorithms and artificial intelligence (AI) process and analyze large datasets to identify patterns and make predictions. These advanced techniques enhance trading strategies' accuracy and efficiency.
- Example: Machine learning algorithms can develop predictive models based on historical data. AI-powered tools analyze patterns and make real-time predictions, helping traders refine their strategies and improve performance.
- Related Link: Learn about machine learning in trading.
Sentiment Analysis
Sentiment analysis tools assess market sentiment by analyzing text data from news articles, social media posts, and other sources. This analysis helps traders gauge market mood and anticipate price movements.
- Example: Sentiment analysis can predict market reactions to news events by evaluating the sentiment of news headlines and social media posts. Traders use this analysis to adjust their strategies based on anticipated market responses.
- Related Link: Explore sentiment analysis tools.
3. Practical Applications of Big Data in Trading
Big data can be applied to various trading strategies and market scenarios, offering valuable insights and enhancing decision-making.
Algorithmic Trading
Developing Algorithms
Algorithmic trading uses computer algorithms to execute trades based on predefined criteria. Big data enables the development of sophisticated algorithms that analyze large datasets to make informed trading decisions.
- Example: Traders create algorithms that analyze market data, news, and social media sentiment to identify trading signals and execute trades automatically. These algorithms improve efficiency and accuracy in trading decisions.
- Related Link: Learn more about algorithmic trading.
High-Frequency Trading (HFT)
High-frequency trading strategies leverage big data to execute a large number of trades within milliseconds. HFT algorithms analyze market data in real-time to capitalize on short-term opportunities.
- Example: HFT strategies use big data to identify and exploit micro-price movements. Algorithms execute trades rapidly based on real-time data, taking advantage of fleeting market opportunities.
- Related Link: Explore high-frequency trading.
Risk Management
Predictive Analytics
Predictive analytics uses big data to forecast potential risks and assess market conditions. By analyzing historical data and identifying patterns, traders can develop risk management strategies to mitigate potential losses.
- Example: Predictive models estimate the likelihood of adverse market movements, helping traders adjust their risk management strategies. Predictive analytics provides insights into potential risks and enables proactive risk mitigation.
- Related Link: Learn about predictive analytics.
Portfolio Optimization
Big data aids in optimizing trading portfolios by analyzing asset correlations, risk factors, and performance metrics. This analysis helps traders construct portfolios that balance risk and return.
- Example: Traders use big data to optimize asset allocation and diversify portfolios. Analysis of historical data and performance metrics helps identify the optimal mix of assets to achieve desired risk-return profiles.
- Related Link: Explore portfolio optimization.
4. Challenges and Solutions
Despite its benefits, harnessing big data for trading presents challenges that need to be addressed.
Data Quality and Integrity
Ensuring Accuracy
Ensuring the accuracy and reliability of big data is crucial for effective analysis. Inaccurate or incomplete data can lead to flawed models and erroneous trading decisions.
- Challenge: Poor data quality can undermine the effectiveness of trading strategies. Inaccurate data may lead to incorrect predictions and poor trading decisions.
- Solution: Use reputable data providers and implement data validation processes to ensure accuracy. Regular audits and cleaning of data help maintain its
integrity.
- Related Link: Learn about data quality management.
Data Privacy and Security
Protecting Sensitive Information
Data privacy and security are essential for maintaining trust and avoiding legal issues. Protecting sensitive trading data from breaches and unauthorized access is critical.
- Challenge: Data breaches and unauthorized access can compromise sensitive trading information and violate privacy regulations.
- Solution: Implement robust security protocols and encryption methods to protect data. Stay informed about data protection regulations and ensure compliance.
- Related Link: Explore data privacy and security.
Data Overload
Managing Large Volumes of Data
Dealing with the sheer volume of big data can be overwhelming. Traders need effective strategies for managing and analyzing large datasets to avoid information overload.
- Challenge: Large volumes of data can be challenging to process and analyze efficiently.
- Solution: Use data management tools and techniques to filter and prioritize relevant information. Employ advanced analytics tools to extract meaningful insights from large datasets.
- Related Link: Learn about data management strategies.
Conclusion
Harnessing big data offers significant advantages for traders looking to improve their decision-making and enhance trading strategies. By leveraging advanced techniques and tools, traders can gain valuable insights, optimize performance, and manage risks effectively. Addressing challenges related to data quality, privacy, and overload is crucial for successful implementation.
Tags Directory
View AllLatest
View All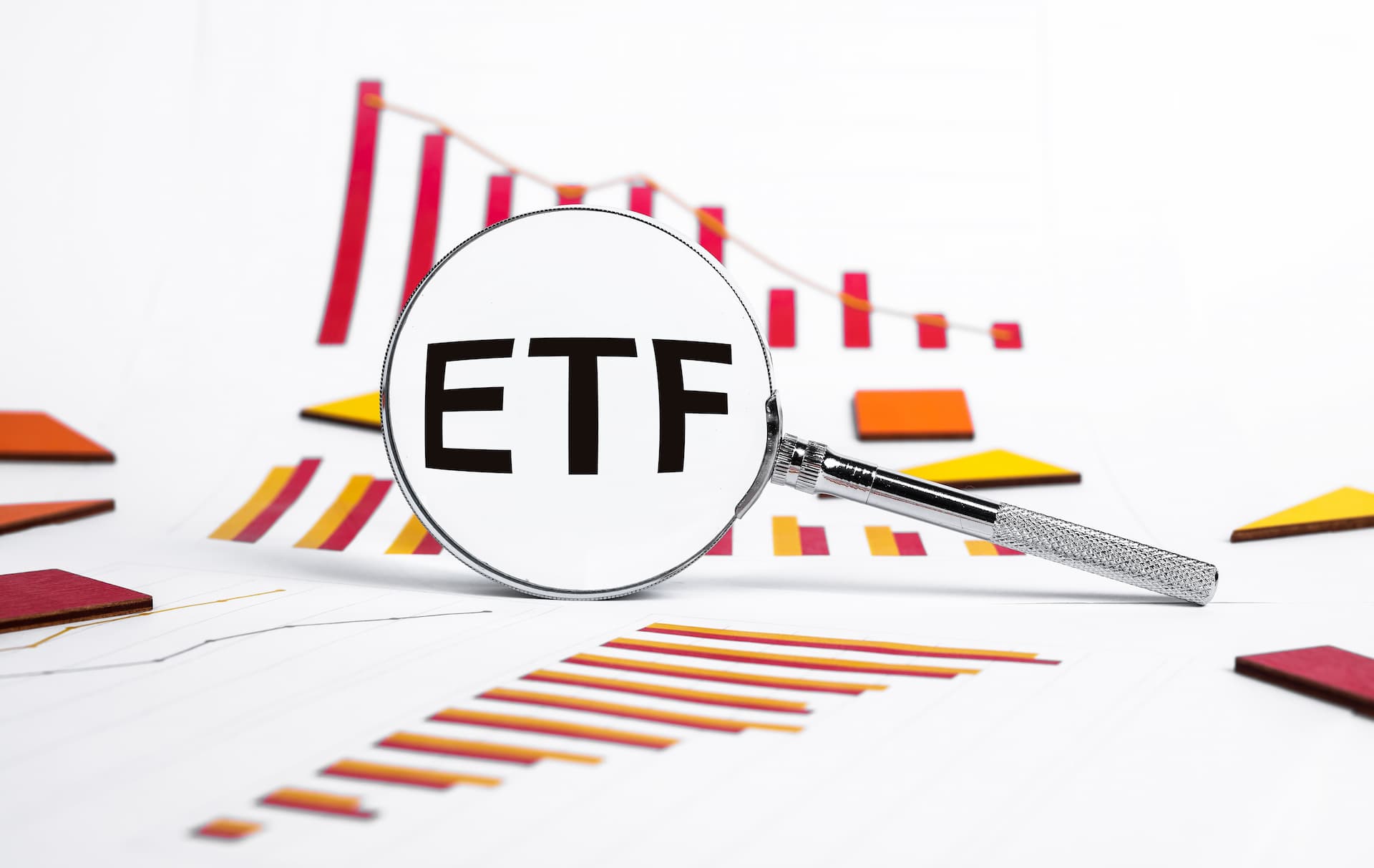
September 9, 2024
4 min
ETF Trading Strategies - 24markets.com
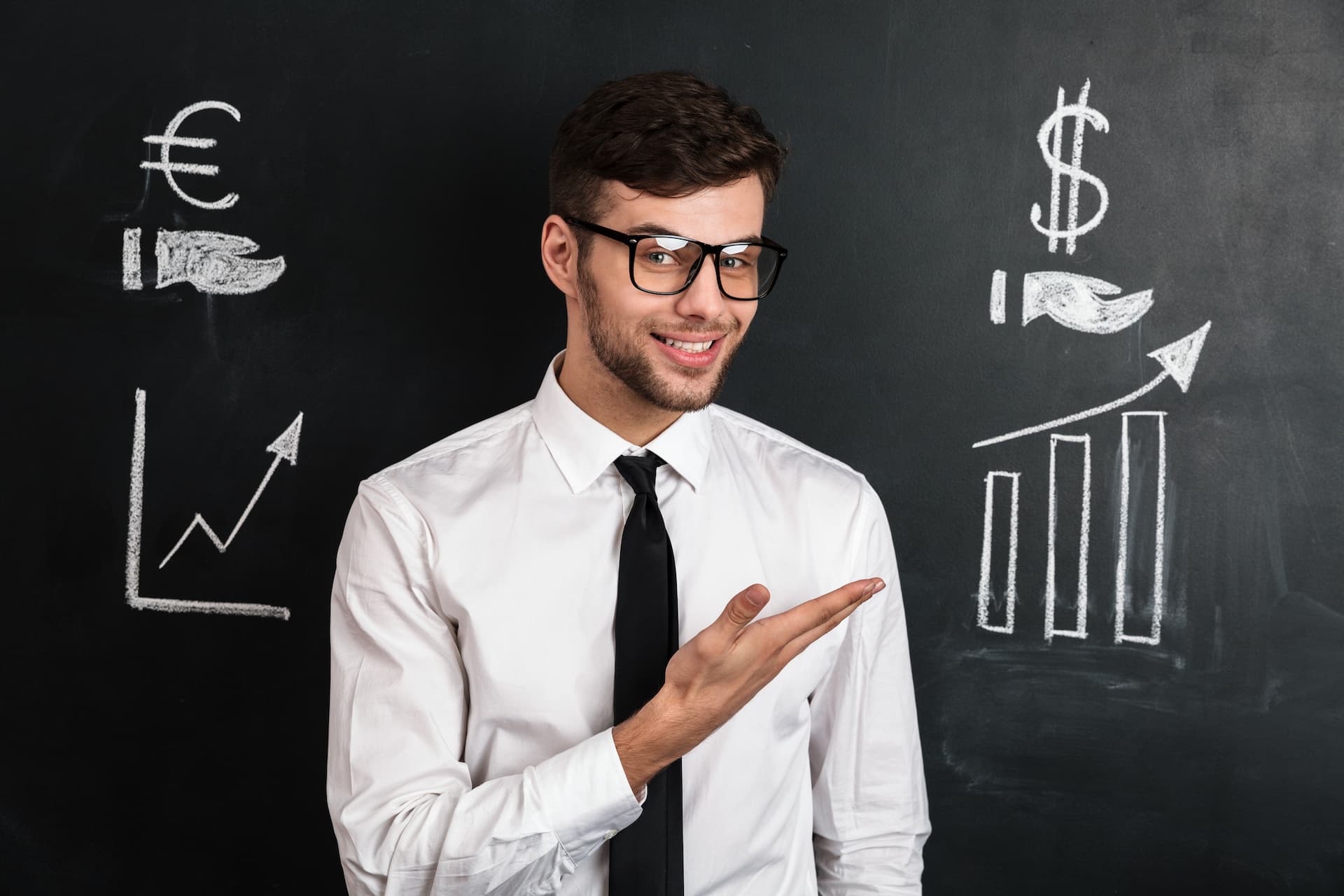
September 9, 2024
6 min
Building Confidence as a Trader - 24markets
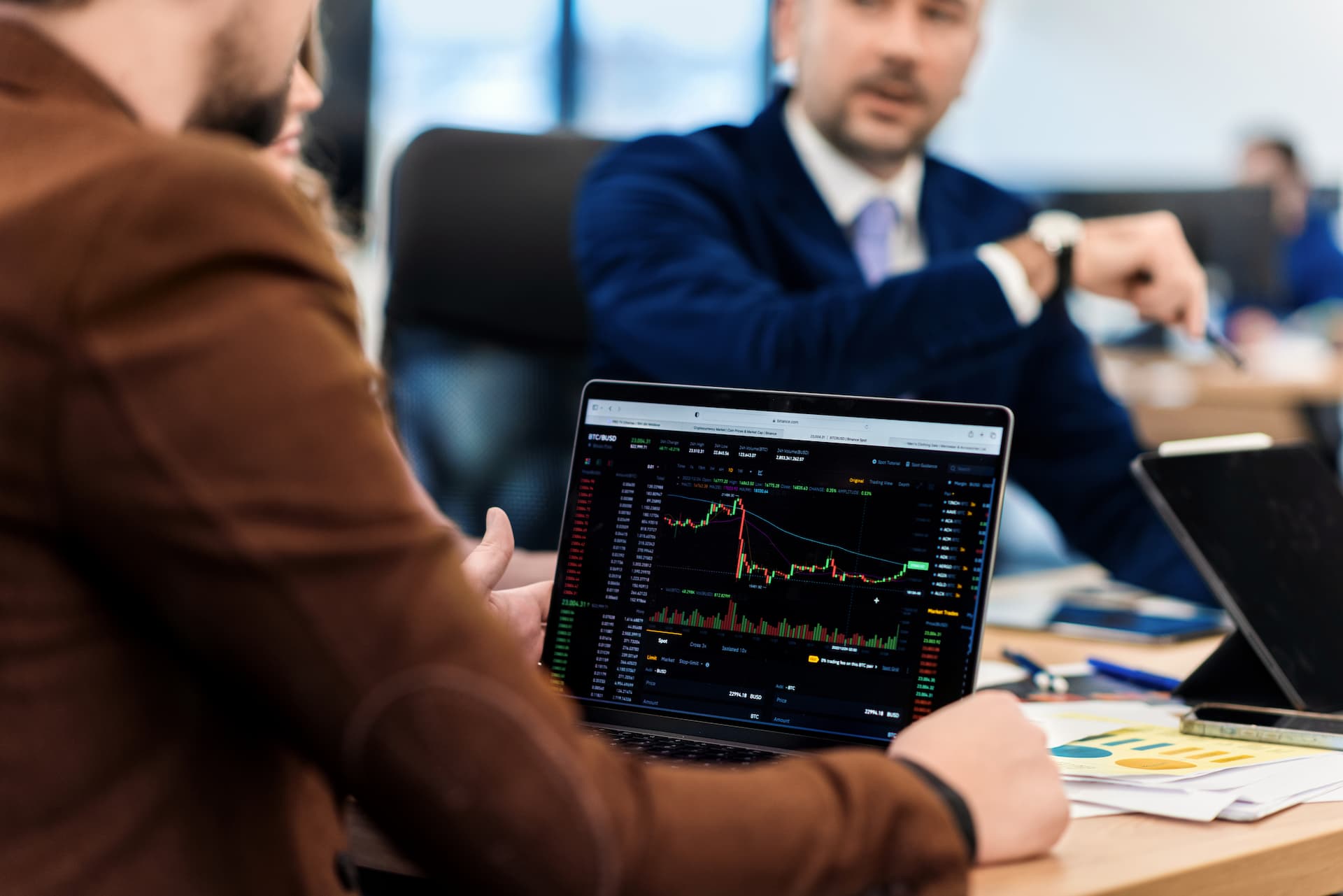
September 9, 2024
3 min
Trading Strategies for Bonds
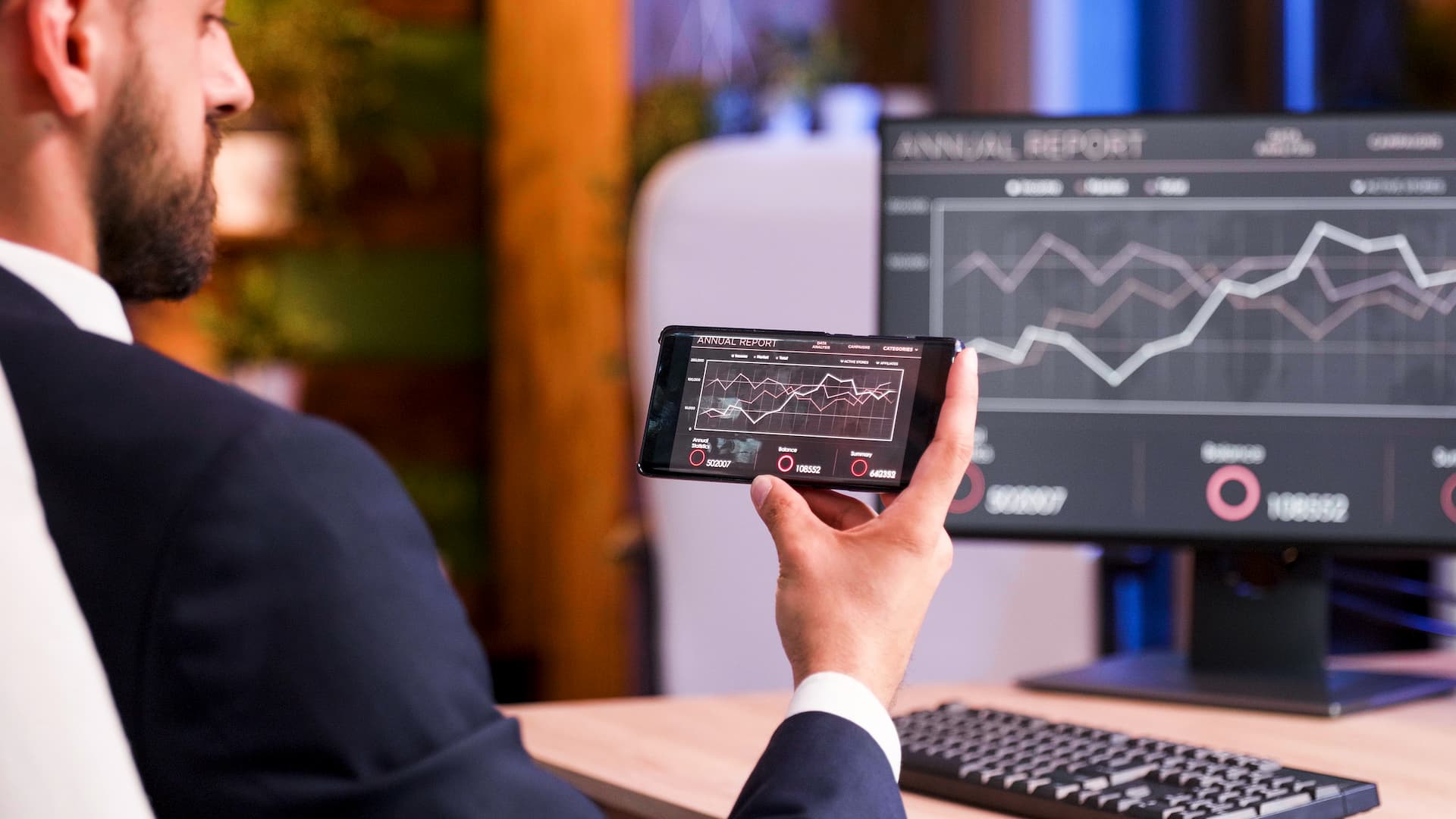
September 9, 2024
4 min
What is Position Sizing? - 24markets
Content
- - Understanding Big Data in Trading
- - Techniques for Harnessing Big Data
- - Practical Applications of Big Data in Trading
- - Challenges and Solutions
- - Conclusion