Calculating Value at Risk (VaR)
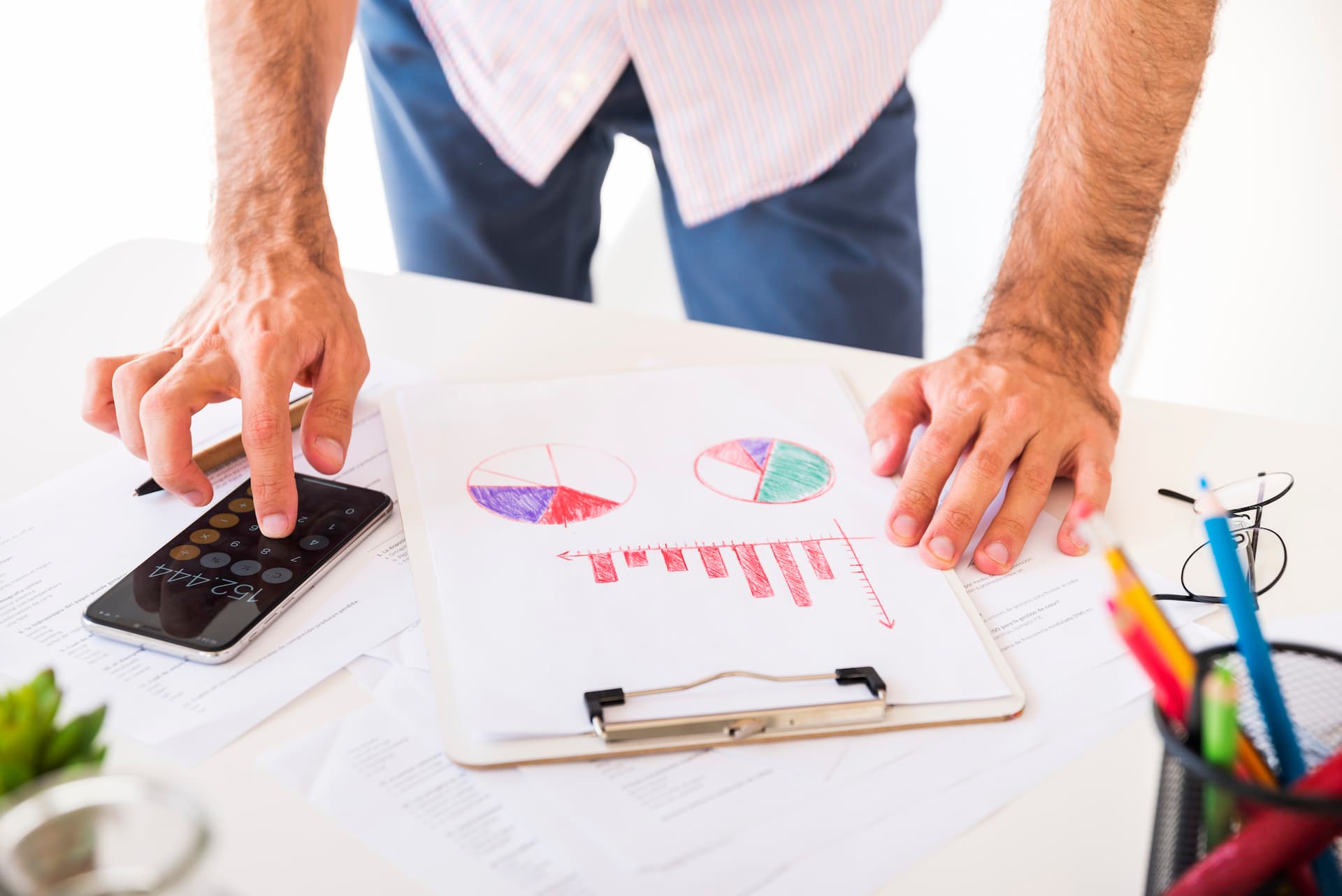
Value at Risk (VaR) is a critical measure used to assess the potential loss in value of a portfolio or investment over a specific period, given a certain confidence level. It provides traders and investors with a quantifiable risk measure to manage their portfolios effectively.
1. Understanding VaR
VaR quantifies the maximum expected loss over a given time period with a specified confidence level. For instance, a 95% confidence level VaR indicates that there is a 95% chance that the portfolio will not lose more than the VaR amount over the specified period.
- Confidence Level: Typically set at 95% or 99%, reflecting the probability that losses will not exceed the VaR estimate. Higher confidence levels (e.g., 99%) provide a more conservative risk measure.
- Time Horizon: VaR can be calculated for various time horizons such as daily, weekly, or monthly, depending on the trading strategy and investment horizon. For more on time horizons and their implications, explore trading basics.
2. Methods to Calculate VaR
a. Historical Simulation
Historical simulation uses historical return data to estimate future risk:
- Step 1: Gather historical price data for the asset or portfolio. Use platforms like 24markets to access historical data.
- Step 2: Compute historical returns over the selected time horizon (e.g., daily returns over the past year). For a detailed guide on calculating returns, visit trading tools.
- Step 3: Sort the returns and find the percentile corresponding to the desired confidence level (e.g., the 5th percentile for a 95% confidence level). For historical simulation techniques, check out risk management strategies.
- Step 4: The VaR is the return at this percentile, indicating the maximum expected loss.
Advantages: Simple to understand and implement; does not require assumptions about return distributions.
Disadvantages: Relies heavily on historical data, which may not predict future risks accurately. For more details on limitations, refer to risk management techniques.
b. Variance-Covariance Method
The variance-covariance method assumes normally distributed returns:
- Step 1: Calculate the mean and standard deviation of returns. Use tools available on 24markets for statistical analysis.
- Step 2: Identify the Z-score for the desired confidence level (e.g., -1.645 for 95% confidence). For more on Z-scores, explore trading tools.
- Step 3: Apply the VaR formula:
VaR=Mean−(Z-Score×Standard Deviation)
Learn more about statistical calculations in trading analytics.
Advantages: Straightforward and computationally efficient; suitable for normally distributed data.
Disadvantages: Assumes normal distribution, which may not capture extreme market movements. For more on these limitations, visit risk management strategies.
c. Monte Carlo Simulation
Monte Carlo simulation generates a range of possible outcomes based on random sampling:
- Step 1: Generate multiple simulated paths of asset returns using statistical models or historical data. Platforms like 24markets provide simulation tools.
- Step 2: Calculate the portfolio value for each simulated path.
- Step 3: Determine the VaR by identifying the percentile of losses in the simulated results.
Advantages: Flexibility to model various distributions and scenarios; can accommodate complex portfolios.
Disadvantages: Computationally intensive and requires robust data and modeling tools. For insights into Monte Carlo methods, visit trading tools.
3. VaR Calculation Example
Here’s an example using the variance-covariance method:
- Mean Return: 0.05 (5%)
- Standard Deviation: 0.10 (10%)
- Confidence Level: 95% (Z-score = -1.645)
VaR=0.05−(−1.645×0.10)=0.05+0.1645=0.2145
In this example, the VaR is 21.45%, indicating a 95% confidence level that the portfolio will not lose more than 21.45% of its value over the specified time horizon.
4. Limitations of VaR
VaR has some notable limitations:
- Historical Data Dependence: Historical simulation relies on past data which may not always reflect future risks. For more on data limitations, visit risk management.
- Assumptions of Normality: The variance-covariance method assumes normal distribution, which may overlook extreme events. For insights on assumptions, refer to trading risks.
- Does Not Measure Extreme Losses: VaR does not account for the magnitude of losses beyond the VaR threshold. Learn more about extreme risk measures in advanced risk management.
5. Using VaR in Risk Management
VaR is often used alongside other risk management tools:
- Stress Testing: Complement VaR with stress testing to evaluate how extreme market conditions affect your portfolio. For more on stress testing, visit trading tools.
- Scenario Analysis: Assess different scenarios to understand potential impacts on your portfolio. Explore scenario analysis techniques in trading strategies.
- Diversification: Use VaR in conjunction with diversification strategies to spread risk across various assets and sectors. For more on diversification, check out portfolio management.
For more detailed information on VaR and risk management strategies, visit 24markets.com.
Tags Directory
View AllLatest
View All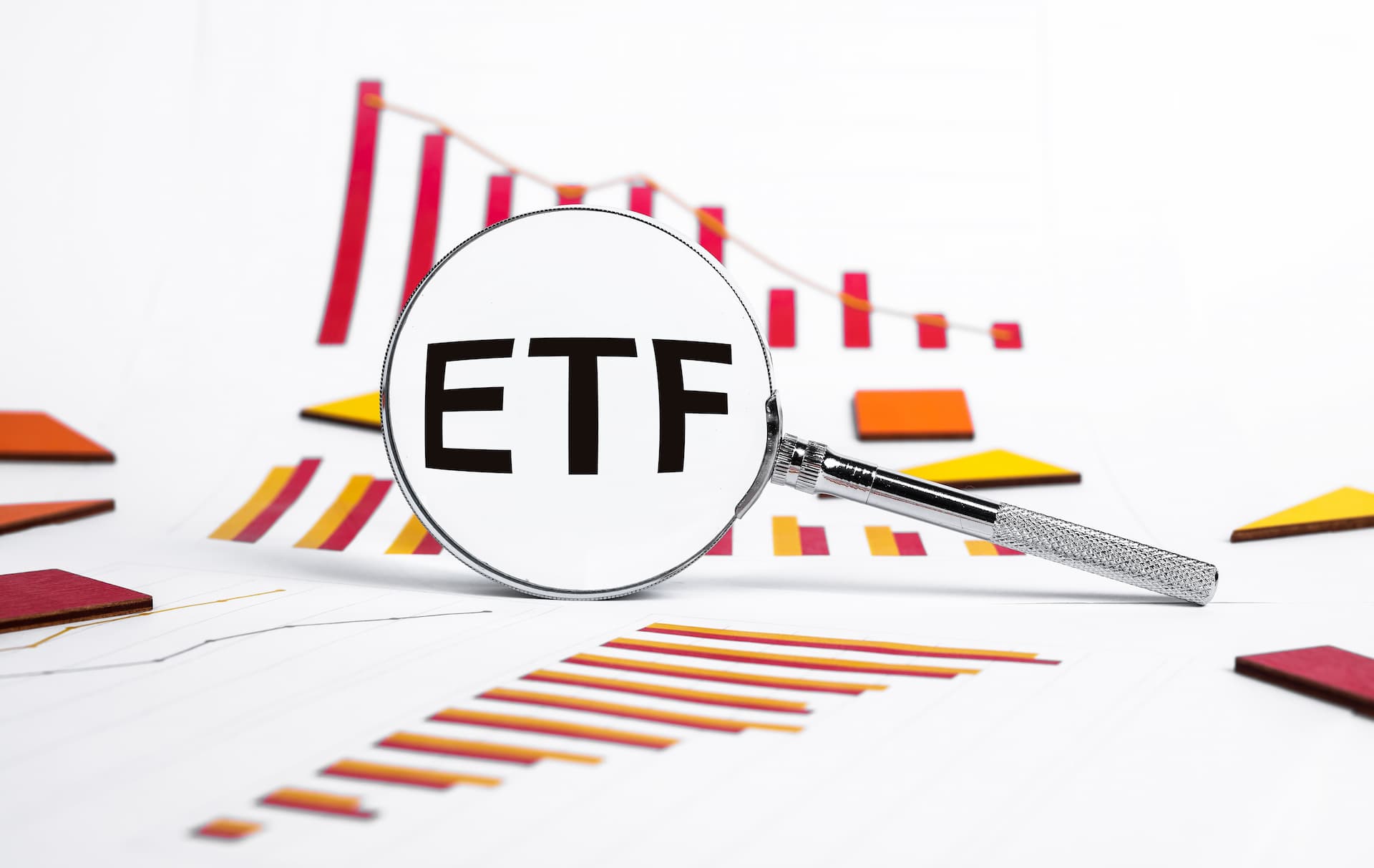
September 9, 2024
4 min
ETF Trading Strategies - 24markets.com
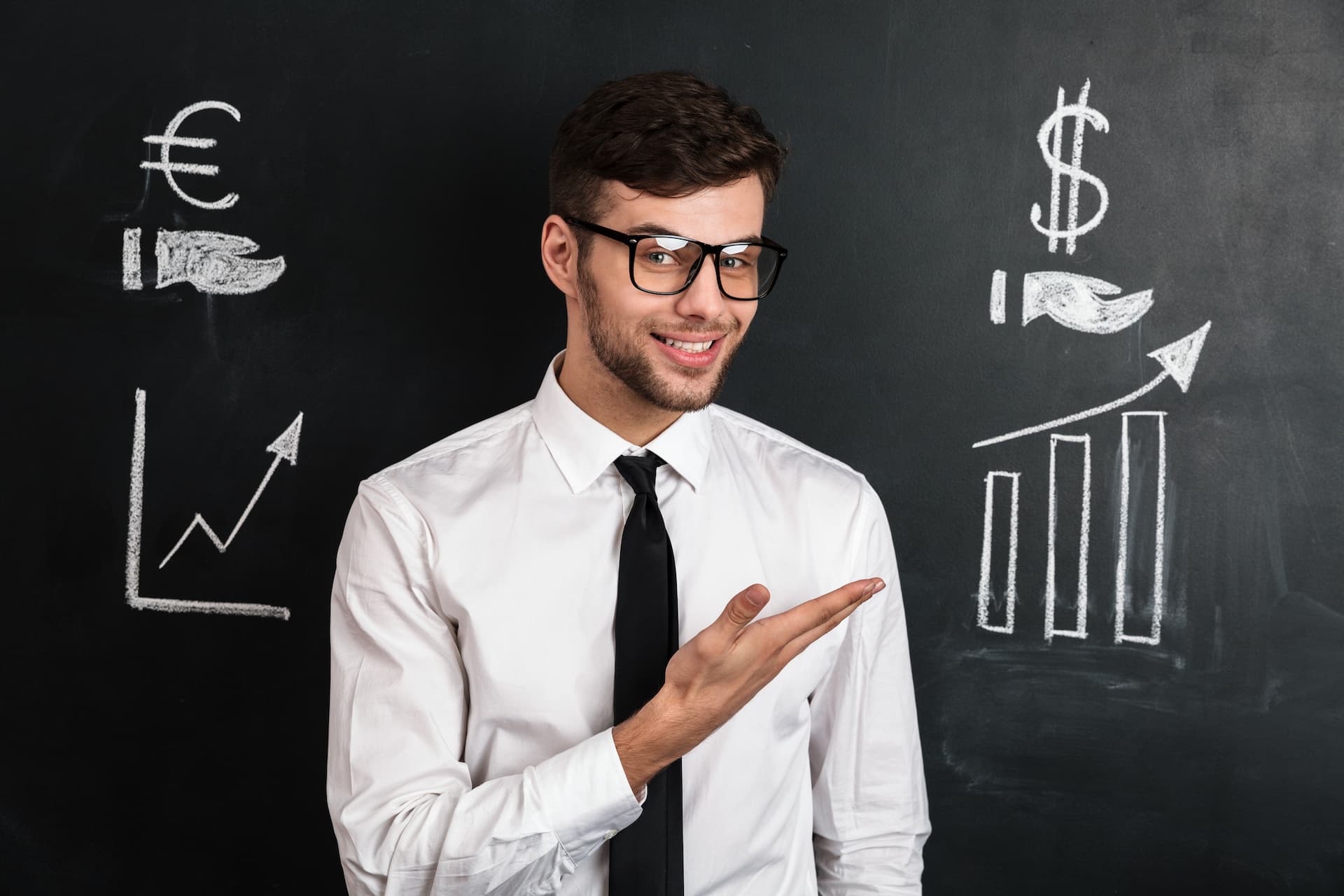
September 9, 2024
6 min
Building Confidence as a Trader - 24markets
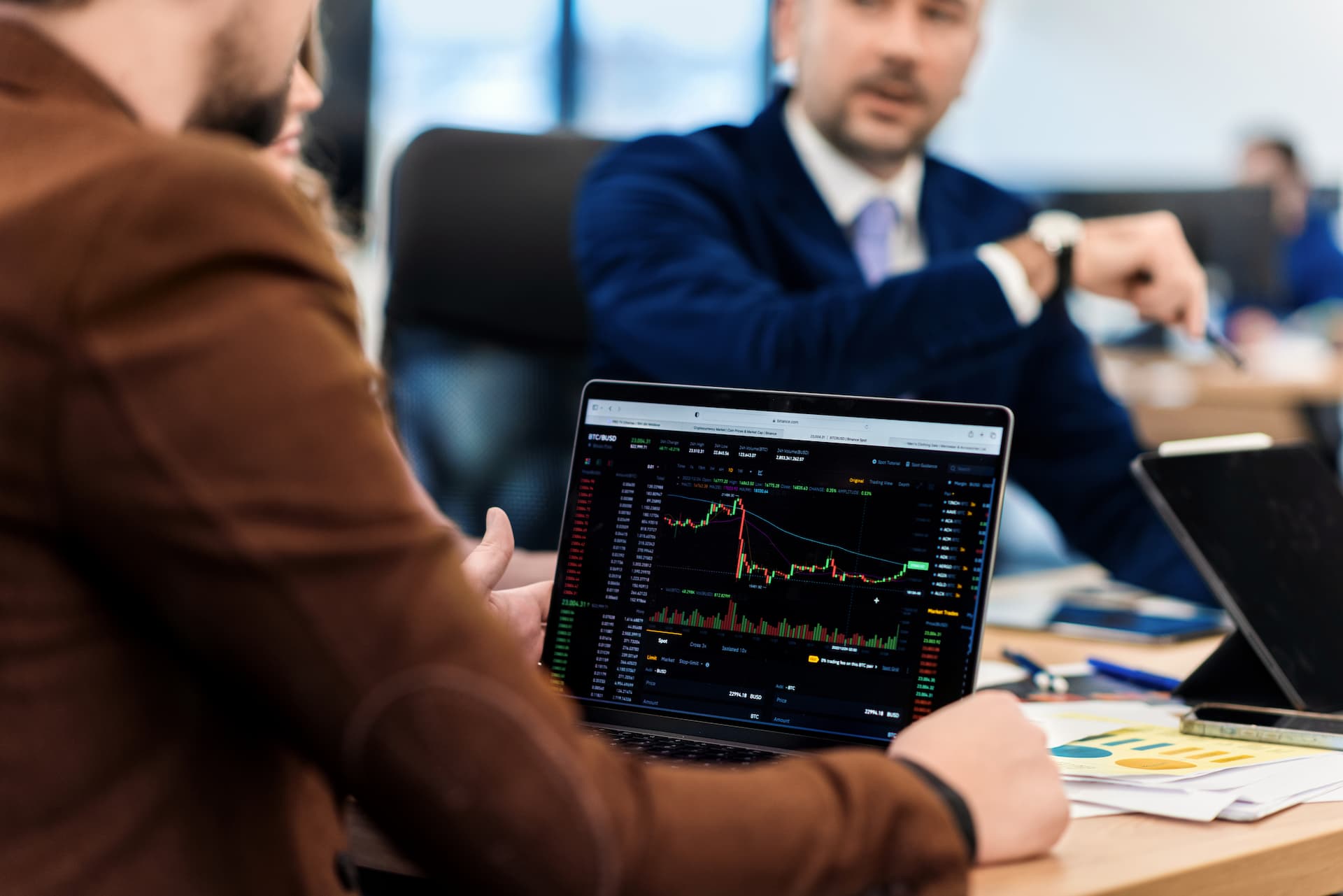
September 9, 2024
3 min
Trading Strategies for Bonds
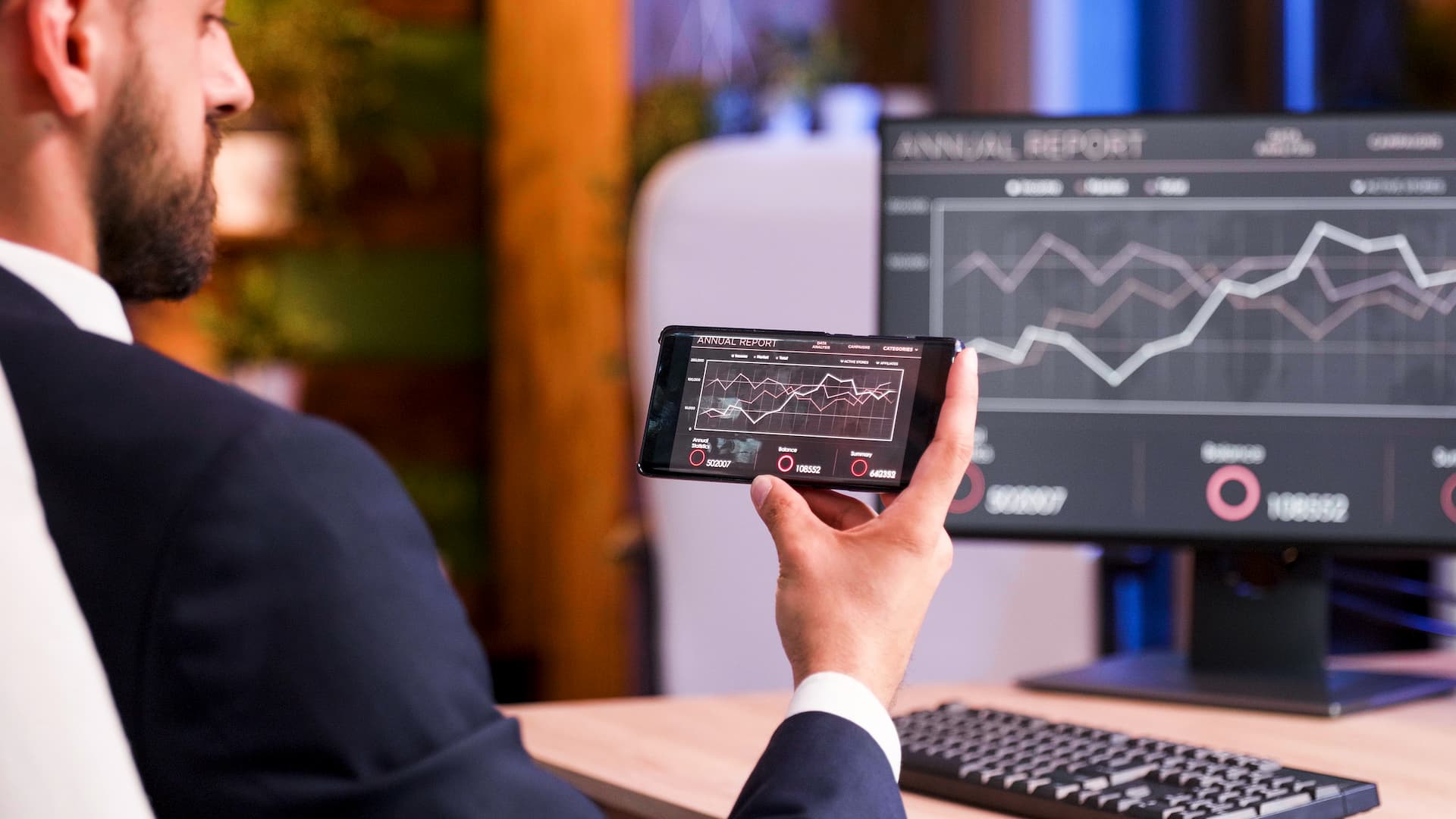
September 9, 2024
4 min
What is Position Sizing? - 24markets
Content
- - Understanding VaR
- - Methods to Calculate VaR
- - a Historical Simulation
- - b VarianceCovariance Method
- - c Monte Carlo Simulation
- - VaR Calculation Example
- - Limitations of VaR
- - Using VaR in Risk Management